Call Center Predictive Modeling
Predictive staffing models help call centers schedule all the agents they need to ensure customer satisfaction while keeping wage costs under control. But times series analysis call center forecasting can do a lot more than just help managers fill out their schedules.
The most basic use of call center forecasting models is to match staffing to expected calls. These forecasting models help managers make sure there are enough agents to handle calls at peak times.
Call center forecasting models to take past data and predict future events. Data for a new call center takes months or years to accumulate, but call centers that have been operating for several years can benefit from predictive modeling. Or managers might simply average data over the past several years to get a ballpark idea of call volume for the current year and add a fudge factor to make sure calls are covered.
All predictive models make assumptions. The point estimate model, for example, assumes that each calendar day correlates to the same calendar in previous years. Having just a few weeks or a few months of data, especially if those weeks or months include seasonal downtime, annual peak demand, or holidays, can make a big difference in the predictive power of the model.
But a more useful approach to call center predictive modeling is to rely on time-series analysis that looks at average time to answer and attempts to identify the factors that influence it. There are off-the-shelf computer programs such as Excel, SPSS, and SAS that can run statistical analyses to find the relative weights of the variables that predict future numbers of calls and their average handling time.
Call Center AI
Artificial intelligence, more commonly known as AI, can do a lot more than simply predict call volume and call handling times. Call center AI software is useful not just for slashing costs but also for improving customer experience.
Using AI to enhance customer experience isn't really optional anymore. The nearly universal use of smartphones, the proliferation of consumer-friendly apps, and the opinion molding accomplished by social media have made providing a good customer experience essential. Call center managers recognize that their needs have moved past just using call center forecasting machine learning to predict staffing needs to recognize how AI is changing call centers with whom they compete for customers.
As a result, in recent years call center AI has given us:
- AI-powered customer assistants. Callers don't have to press 0 and wait for an agent. AI can relieve agents from the majority of calls that are just requesting basic information and allow them to focus their efforts on issues that can't be handled by just giving customers information.
- AI-power call agents. AI can keep track of how many times customers have called in with product issues, or generalized complaints, or threatened to take their business elsewhere. The same program can prompt the call agent with empathetic responses and company offers to keep the customer's business (or not, if the AI recommends letting the customer go).
- Predictive call routing. Some agents have more expertise at certain kinds of caller issues than other agents. AI can route those calls to the most appropriate agents. AI can also evaluate customer personality types and direct calls to agents with the greatest skills in dealing with those personality types.
The US market for call-center AI technology is expected to increase from $800 million in 2019 to just a little under $3 billion by 2024. The demand for AI for call centers is driven by the need to provide customers with the highest-quality call-in experience.
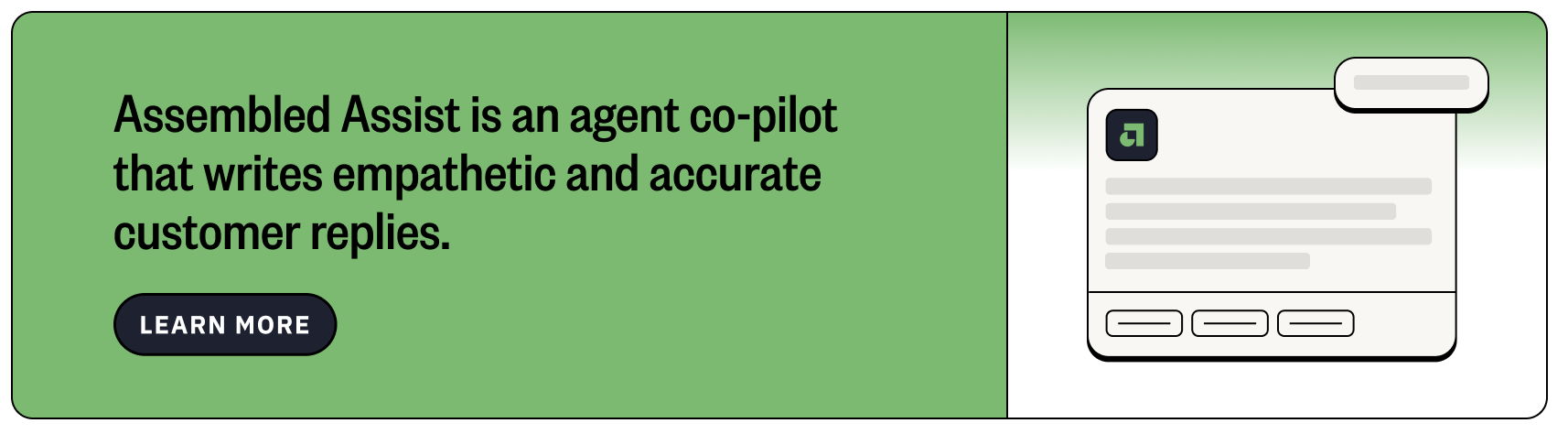
Call Center Analytics Use Cases
McKinsey & Company has helped a number of call centers use predictive modeling. As one of the world's premier call center analytics companies, it has used predictive analytics to reduce AHT (average handle time) by up to 40 percent, reduce call escalation rates by 5 to 20 percent, cut employees costs per call center by up to $5 million, and jump-start service to sales calls ratio by up to 50 percent—at the same time call centers improved employee engagement and customer satisfaction. Their call center analytics use cases show that predictive analytics can transform a call center from a service to a strategic differentiator that can help a company stand out in the marketplace.
One call center analytics case study from McKinsey concerned a company that used text and speech analytics to reduce average call handling time by 40 percent. The company analyzed a specific kind of problem incident by analyzing not just the chat between customers and call center agents but also social media channels, surveys, feedback forms, warranty claims, and other text sources related to the problem. With that information, the company was able to redesign questions to understand customer problems better and to eliminate unnecessary steps, and standardize customer service agent responses.
In another call center analytics case study by McKinsey looked at millions of customer data points to reduce customer call volume. The company was receiving a high volume of repeat calls. For every 100 customer problems, the call center was receiving 160 calls. The analysis identified customers who would call about minor issues, such as a status update on an issue that had already been resolved, and customers who would try a different agent if they didn't get the resolution they wanted with their first call. Analytics also identified agents who were spending too much time on minor issues.
A third call center analytics case study by the well-known consulting firm proactively empowered a case study to become resilient to outages. The typical call center suffers five major disruptions to calls every year, with 25 to 30 disruptions to call queues. It can take a week to recover from a call outage, and 10 to 30 percent of customers can be lost in the process.
Workforce-management advanced analytics enabled a call center to prioritize the actions they need to take when the weather keeps call agents from coming in, or the call center suffers a power outage, or a server goes down.
McKinsey & Company also helped one company create a virtual sales coach that unlocked new revenues by predicting the products a customer would buy next and giving call agents a script to encourage upgrades and purchases. These analytics locate the conversation the agent used in a previous successful sales call with the customer so the agent can use the same approach again.
Contact Center Insights
Call center analytics can produce an astonishing range of actionable insights. Here are just a few:
- A simple fact of sales is that it is a lot more expensive to acquire a new customer than it is to renew contracts and orders for existing customers. Predictive analytics can locate the factors in call agent contact that are most closely related to whether existing customers renew or order again.
- Predictive analytics can match your call center's best closers with your best leads.
- Top-performing agents can be given the calls most likely to result in revenue.
- Predictive analytics can be turned around to analyze agents instead of customers.
- AI can identify the agents that get the most positive feedback or who close the most sales and find out what it is that they do that accounts for their success.
- AI can give your call agents real-time feedback about whether they are meeting their goals. They can see their average handling time and other key performance indicators.
- Text analytics, speech analytics, and structured data fields can be combined to understand why customers cancel services or fail to make additional purchases. Then call agents can be given tools to retain customer relationships based on real-time analysis of text, speech, and other data.
Call center data science gives companies a way to measure the success of marketing campaigns beyond just counting the number of calls. AI combined with speech recognition can measure the frequency of phrases like "I'm calling about the offer I saw on TV the other day" or "I was searching for something like your product online and your page showed up in the top of the results."
Companies that use call center data science can focus their efforts where they are most likely to result in sales, keeping agents happier while adding to the bottom line.
Contact Center Analytics Solutions
What do all of these capabilities tell us about the real answer to the question "What is contact center analytics?"
Here's the key point that call center managers need to take away:
- Contact center analytics solutions aren't just about what your call center is doing right now.
- Contact center analytics are also about what your call center can achieve in the future.
Customer service leads need more than just real-time information to keep the needs of the customer first. They also need advanced analytics to help them know what their customers will need and want next. Advanced analytics reduce costs, increase revenues, and keep customers happier by mining both inward-facing and call center customer data.
What do call centers need to take contact center analytics solutions to the next level? They need:
- Integrated data across sales and service channels. It's not enough to have money-making sales and service silos. It's essential to be able to see the big picture with historical and predictive data.
- Knowing what to do with analytical insights. It's not enough to fix a problem with analytics data. It's also necessary to turn data into changes in procedure and policy that increase revenues and customer satisfaction.
- Agile organization. Managers need to focus on changes they can implement with an acceptable level of disruption to the call center's routine.
- Platforms everyone can understand. Data need to be accessible for all stakeholders, preferably in a single data lake.
- A culture of objective decision-making. Managers need to make decisions on the basis of data rather than on the basis of gut instinct.
Call Center Optimization Model
Modern analytics tools put a viable call center optimization model in reach for the management of every call center. Analytics don't just answer questions like call center seating optimization and predicting call center performance with machine learning. Modern call center analytics create a call center optimization model that helps the center stand out from its competition with superior customer service and lower operating costs.